K-mer counting tool
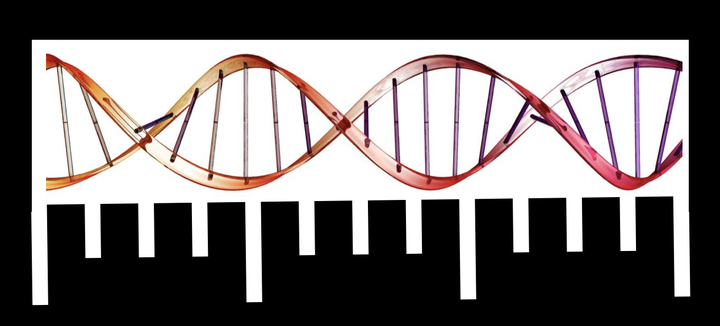
This post contains a short analysis of 4 FASTA files each containing a single nucleotide sequence. The goal of the analysis is to determine if the sequences have a balanced number of k-mer (4-mer) counts. The post also includes instructions on how to use the kmer_counting_tool.R script as a command line tool that lets the user select an input FASTA file and a k-mer length, and returns a file counts for each kmer sequence.
Import the FASTA files with the Biostrings package
The nucleotide sequences are imported into R as DNAStringSet objects but I will convert them into a data frame and then slice the sequences into k-mers.
#101010 black
#FDF7F7 white
library("Biostrings")
library(tidyverse)
library(gt)
library(patchwork)
theme_set(theme_light())
exp1 <- readDNAStringSet('takehome/challenge1/experiment1.fasta')
exp1
## DNAStringSet object of length 1:
## width seq names
## [1] 10000 GTATTTACAGCAAAATTATATAT...ACTGCCATTTTGTCTTTTATGAG
exp2 <- readDNAStringSet('takehome/challenge1/experiment2.fasta')
exp3 <- readDNAStringSet('takehome/challenge1/experiment3.fasta')
exp4 <- readDNAStringSet('takehome/challenge1/experiment4.fasta')
#exp5 = readDNAStringSet("nonstandard_nucs.fasta")
fasta_files = list(exp1, exp2, exp3, exp4)
#Function:
#convert_to_tibble()
convert_to_tibble <- function(s) {
seq_name <- names(s)
sequence <- paste(s)
df <- tibble(seq_name, sequence)
}
fasta_df <- map_df(fasta_files, convert_to_tibble)
fasta_df$seq_name = c('exp1', 'exp2', 'exp3', 'exp4')
# https://github.com/tidyverse/stringr/issues/292
str_dice <- function(s, length) { # does not return list
L <- str_length(s)
str_sub(s, start=seq(1L,L-length+1,length), end=seq(length,L,length))
}
#Function:
#kmer_len()
kmer_len <- function(tbl) {
tbl %>%
str_dice(length = 4) %>%
tibble() %>%
rename(kmer = ".") %>%
count(kmer, sort = T, name = 'kmer_count') %>%
mutate(kmer = fct_reorder(kmer, kmer_count)) %>%
mutate(standard_nucs = str_detect(kmer, "[non_stand_nucs]", negate = T))
}
Table of K-mer counts by Experiment
This table is similar to the tab separated output file that is produced from the kmer_counting_tool.R
where Exp1 = sequence 1 counts, etc.
seq1 <- fasta_df$sequence[1] %>%
kmer_len() %>%
mutate(experiment = 'Exp1_counts')
seq2 <- fasta_df$sequence[2] %>%
kmer_len() %>%
mutate(experiment = 'Exp2_counts')
seq3 <- fasta_df$sequence[3] %>%
kmer_len() %>%
mutate(experiment = 'Exp3_counts')
seq4 <- fasta_df$sequence[4] %>%
kmer_len() %>%
mutate(experiment = 'Exp4_counts')
sequences <- bind_rows(seq1, seq2, seq3, seq4)
sequences %>%
pivot_wider(names_from = experiment, values_from = kmer_count) %>%
gt() %>%
tab_header(title = md("**Kmer counts by experiment**")) %>%
tab_options(container.height = 400,
container.overflow.y = TRUE,
heading.background.color = "#EFFBFC",
table.width = "75%",
column_labels.background.color = "black",
table.font.color = "black") %>%
tab_style(style = list(cell_fill(color = "Grey")),
locations = cells_body())
Kmer counts by experiment | |||||
---|---|---|---|---|---|
kmer | standard_nucs | Exp1_counts | Exp2_counts | Exp3_counts | Exp4_counts |
ATAA | TRUE | 77 | 45 | 22 | 10 |
TAAT | TRUE | 73 | 37 | 19 | 8 |
ATAT | TRUE | 66 | 38 | 24 | 9 |
TATT | TRUE | 66 | 28 | 27 | 11 |
AAAT | TRUE | 65 | 30 | 22 | 14 |
AATT | TRUE | 65 | 36 | 15 | 11 |
TTTT | TRUE | 63 | 29 | 28 | 9 |
TAAA | TRUE | 60 | 30 | 24 | 9 |
ATTT | TRUE | 58 | 33 | 24 | 7 |
TTAT | TRUE | 57 | 48 | 23 | 8 |
AATA | TRUE | 55 | 39 | 15 | 7 |
AAAA | TRUE | 53 | 45 | 25 | 6 |
ATTA | TRUE | 53 | 32 | 18 | 7 |
TTTA | TRUE | 53 | 36 | 23 | 10 |
TATA | TRUE | 50 | 34 | 17 | 5 |
TTAA | TRUE | 49 | 37 | 21 | 9 |
GATT | TRUE | 26 | 14 | 12 | 8 |
GTTT | TRUE | 25 | 21 | 19 | 15 |
TACT | TRUE | 24 | 14 | 16 | 12 |
ACTA | TRUE | 22 | 19 | 15 | 7 |
ATAC | TRUE | 22 | 19 | 11 | 9 |
ATGA | TRUE | 22 | 17 | 13 | 6 |
TACA | TRUE | 21 | 19 | 10 | 14 |
TGTT | TRUE | 21 | 13 | 12 | 13 |
ACTT | TRUE | 20 | 14 | 11 | 11 |
CTAA | TRUE | 20 | 16 | 16 | 10 |
AAGT | TRUE | 19 | 10 | 14 | 16 |
CATA | TRUE | 19 | 15 | 18 | 10 |
GAAA | TRUE | 19 | 14 | 10 | 11 |
GTAT | TRUE | 19 | 13 | 16 | 10 |
TAAC | TRUE | 19 | 14 | 15 | 12 |
TCAA | TRUE | 19 | 16 | 14 | 12 |
ACAT | TRUE | 18 | 21 | 19 | 8 |
AGTT | TRUE | 18 | 22 | 19 | 9 |
CTAT | TRUE | 18 | 15 | 14 | 5 |
TTGT | TRUE | 18 | 15 | 12 | 8 |
AACA | TRUE | 17 | 12 | 15 | 9 |
AGAT | TRUE | 17 | 18 | 15 | 8 |
CATT | TRUE | 17 | 22 | 13 | 6 |
CTTT | TRUE | 17 | 12 | 8 | 8 |
TAAG | TRUE | 17 | 22 | 18 | 7 |
TAGA | TRUE | 17 | 15 | 15 | 7 |
TTTG | TRUE | 17 | 20 | 14 | 10 |
AAAC | TRUE | 16 | 17 | 10 | 13 |
AAAG | TRUE | 16 | 18 | 12 | 9 |
AACT | TRUE | 16 | 16 | 21 | 11 |
ACAA | TRUE | 16 | 16 | 12 | 5 |
ATCT | TRUE | 16 | 12 | 16 | 10 |
GTAA | TRUE | 16 | 11 | 11 | 13 |
GTTA | TRUE | 16 | 14 | 11 | 11 |
TGAA | TRUE | 16 | 20 | 12 | 14 |
TGTA | TRUE | 16 | 17 | 13 | 12 |
TTAG | TRUE | 16 | 16 | 9 | 6 |
TTCT | TRUE | 16 | 21 | 9 | 13 |
AAGA | TRUE | 15 | 22 | 16 | 9 |
CAAA | TRUE | 15 | 23 | 11 | 14 |
TCTA | TRUE | 15 | 12 | 14 | 12 |
TGAT | TRUE | 15 | 12 | 13 | 9 |
TTAC | TRUE | 15 | 13 | 11 | 7 |
TTGA | TRUE | 15 | 14 | 10 | 10 |
ATTG | TRUE | 14 | 14 | 16 | 9 |
CAAT | TRUE | 14 | 16 | 16 | 10 |
CTTA | TRUE | 14 | 25 | 13 | 7 |
GAAT | TRUE | 14 | 19 | 9 | 9 |
GATA | TRUE | 14 | 17 | 18 | 10 |
TATC | TRUE | 14 | 12 | 18 | 7 |
TCTT | TRUE | 14 | 15 | 13 | 13 |
TTTC | TRUE | 14 | 27 | 12 | 6 |
AGTA | TRUE | 13 | 15 | 10 | 12 |
TAGT | TRUE | 13 | 12 | 15 | 7 |
TATG | TRUE | 13 | 23 | 18 | 10 |
AATG | TRUE | 12 | 14 | 12 | 10 |
AGAA | TRUE | 12 | 26 | 16 | 10 |
ATCA | TRUE | 12 | 20 | 10 | 9 |
ATTC | TRUE | 12 | 14 | 15 | 14 |
TCAT | TRUE | 12 | 20 | 14 | 13 |
AATC | TRUE | 11 | 17 | 11 | 9 |
ATAG | TRUE | 11 | 18 | 14 | 9 |
TTCA | TRUE | 11 | 11 | 11 | 12 |
ATGT | TRUE | 10 | 18 | 10 | 10 |
CATC | TRUE | 9 | 13 | 8 | 11 |
CGTA | TRUE | 9 | 6 | 10 | 13 |
CTCT | TRUE | 9 | 8 | 9 | 7 |
GTCA | TRUE | 9 | 3 | 6 | 6 |
GTCT | TRUE | 9 | 4 | 5 | 10 |
CAAC | TRUE | 8 | 5 | 11 | 9 |
CCAA | TRUE | 8 | 5 | 8 | 15 |
CGAA | TRUE | 8 | 10 | 7 | 11 |
TCCT | TRUE | 8 | 9 | 16 | 9 |
TGAC | TRUE | 8 | 9 | 14 | 7 |
AGTG | TRUE | 7 | 14 | 4 | 10 |
ATCC | TRUE | 7 | NA | 12 | 14 |
GAAG | TRUE | 7 | 7 | 6 | 7 |
GACA | TRUE | 7 | 4 | 12 | 9 |
GAGT | TRUE | 7 | 4 | 10 | 9 |
GCAA | TRUE | 7 | 8 | 7 | 13 |
GTGA | TRUE | 7 | 10 | 10 | 12 |
TCAG | TRUE | 7 | 7 | 9 | 6 |
AAGC | TRUE | 6 | 6 | 6 | 7 |
AAGG | TRUE | 6 | 1 | 4 | 13 |
ACAC | TRUE | 6 | 12 | 7 | 8 |
ACAG | TRUE | 6 | 9 | 6 | 6 |
ACCA | TRUE | 6 | 2 | 9 | 9 |
AGGT | TRUE | 6 | 7 | 9 | 11 |
CAAG | TRUE | 6 | 7 | 10 | 7 |
GAAC | TRUE | 6 | 11 | 9 | 7 |
GCAT | TRUE | 6 | 6 | 13 | 15 |
TACC | TRUE | 6 | 4 | 8 | 14 |
ACGT | TRUE | 5 | 5 | 10 | 14 |
ACTG | TRUE | 5 | 7 | 11 | 7 |
AGAC | TRUE | 5 | 5 | 11 | 17 |
AGCT | TRUE | 5 | 11 | 9 | 8 |
ATGG | TRUE | 5 | 3 | 13 | 17 |
CACT | TRUE | 5 | 7 | 7 | 13 |
CAGT | TRUE | 5 | 4 | 6 | 12 |
CATG | TRUE | 5 | 8 | 12 | 5 |
CTGT | TRUE | 5 | 13 | 11 | 10 |
GACT | TRUE | 5 | 14 | 5 | 11 |
GATG | TRUE | 5 | 9 | 11 | 16 |
GGTA | TRUE | 5 | 4 | 11 | 8 |
TAGC | TRUE | 5 | 4 | 14 | 10 |
TCAC | TRUE | 5 | 3 | 10 | 6 |
TGCT | TRUE | 5 | 5 | 7 | 7 |
TGTC | TRUE | 5 | 6 | 13 | 15 |
AACG | TRUE | 4 | 10 | 9 | 9 |
ACGA | TRUE | 4 | 8 | 6 | 6 |
AGGA | TRUE | 4 | 12 | 11 | 9 |
CGTT | TRUE | 4 | 6 | 10 | 12 |
CTTC | TRUE | 4 | 5 | 12 | 12 |
GCTT | TRUE | 4 | 3 | 10 | 8 |
GGTT | TRUE | 4 | 8 | 10 | 10 |
GTTC | TRUE | 4 | 12 | 12 | 12 |
TCTG | TRUE | 4 | 5 | 5 | 13 |
TGAG | TRUE | 4 | 8 | 5 | 10 |
TGCA | TRUE | 4 | 8 | 6 | 5 |
TTCG | TRUE | 4 | 6 | 9 | 10 |
ACTC | TRUE | 3 | 8 | 5 | 15 |
AGAG | TRUE | 3 | 4 | 5 | 5 |
AGCA | TRUE | 3 | 11 | 7 | 10 |
AGTC | TRUE | 3 | 6 | 14 | 8 |
CACA | TRUE | 3 | 6 | 5 | 8 |
CACG | TRUE | 3 | 2 | 5 | 11 |
CCTA | TRUE | 3 | 7 | 9 | 11 |
CCTT | TRUE | 3 | 10 | 5 | 12 |
CGGA | TRUE | 3 | 3 | 5 | 6 |
CTAC | TRUE | 3 | 8 | 9 | 10 |
CTGA | TRUE | 3 | 5 | 4 | 11 |
GACG | TRUE | 3 | 5 | 4 | 12 |
GATC | TRUE | 3 | 9 | 14 | 8 |
GCCA | TRUE | 3 | 4 | 8 | 10 |
GGAT | TRUE | 3 | 8 | 7 | 13 |
GGCA | TRUE | 3 | 5 | 7 | 6 |
GGGT | TRUE | 3 | 1 | 7 | 9 |
GTAC | TRUE | 3 | 8 | 9 | 8 |
GTAG | TRUE | 3 | 5 | 9 | 7 |
TAGG | TRUE | 3 | 4 | 6 | 7 |
TCGA | TRUE | 3 | 7 | 4 | 12 |
TCTC | TRUE | 3 | 7 | 12 | 13 |
TGGT | TRUE | 3 | 7 | 11 | 9 |
TGTG | TRUE | 3 | 6 | 8 | 13 |
TTGG | TRUE | 3 | 6 | 10 | 9 |
AACC | TRUE | 2 | 6 | 12 | 12 |
ACCT | TRUE | 2 | 6 | 8 | 10 |
ACGG | TRUE | 2 | 1 | 6 | 7 |
ATCG | TRUE | 2 | 7 | 8 | 10 |
ATGC | TRUE | 2 | 7 | 10 | 11 |
CACC | TRUE | 2 | 2 | 4 | 14 |
CAGA | TRUE | 2 | 9 | 5 | 11 |
CCAT | TRUE | 2 | 1 | 14 | 8 |
CGAT | TRUE | 2 | 7 | 5 | 13 |
CGCC | TRUE | 2 | 1 | 4 | 6 |
CTAG | TRUE | 2 | 10 | 7 | 6 |
CTGC | TRUE | 2 | 1 | 6 | 12 |
CTTG | TRUE | 2 | 4 | 8 | 13 |
GCAC | TRUE | 2 | 5 | 6 | 10 |
GCCT | TRUE | 2 | 4 | 10 | 12 |
GCTA | TRUE | 2 | 4 | 6 | 12 |
GGAA | TRUE | 2 | 7 | 9 | 12 |
GGAC | TRUE | 2 | 7 | 12 | 12 |
GTCC | TRUE | 2 | 3 | 6 | 8 |
GTGT | TRUE | 2 | 8 | 9 | 11 |
GTTG | TRUE | 2 | 11 | 10 | 9 |
TACG | TRUE | 2 | 9 | 8 | 9 |
TCCA | TRUE | 2 | 9 | 18 | 10 |
TCCG | TRUE | 2 | 1 | 9 | 7 |
TCGC | TRUE | 2 | 1 | 6 | 9 |
TGCG | TRUE | 2 | 3 | 5 | 8 |
TTCC | TRUE | 2 | 2 | 7 | 10 |
TTGC | TRUE | 2 | 8 | 6 | 9 |
ACCG | TRUE | 1 | 1 | 8 | 12 |
ACGC | TRUE | 1 | 4 | 6 | 6 |
AGCC | TRUE | 1 | 3 | 10 | 6 |
AGGC | TRUE | 1 | NA | 5 | 7 |
CAGC | TRUE | 1 | 4 | 4 | 6 |
CCAC | TRUE | 1 | 2 | 3 | 12 |
CCAG | TRUE | 1 | 1 | 10 | 11 |
CCCC | TRUE | 1 | NA | 5 | 5 |
CCCT | TRUE | 1 | 3 | 8 | 8 |
CCTC | TRUE | 1 | 2 | 6 | 12 |
CGAC | TRUE | 1 | 2 | 6 | 11 |
CGCT | TRUE | 1 | 1 | 4 | 12 |
CGGT | TRUE | 1 | 3 | 2 | 11 |
CTCA | TRUE | 1 | 7 | 6 | 9 |
CTCC | TRUE | 1 | 2 | 2 | 7 |
CTCG | TRUE | 1 | 3 | 5 | 8 |
GACC | TRUE | 1 | NA | 2 | 6 |
GAGA | TRUE | 1 | 4 | 9 | 11 |
GAGC | TRUE | 1 | 2 | 9 | 9 |
GCGA | TRUE | 1 | NA | 6 | 12 |
GCGT | TRUE | 1 | 3 | 4 | 7 |
GCTC | TRUE | 1 | 1 | 5 | 13 |
GGAG | TRUE | 1 | 1 | 3 | 9 |
GGCC | TRUE | 1 | 1 | 5 | 7 |
GGGC | TRUE | 1 | 2 | 6 | 7 |
TCGG | TRUE | 1 | NA | 5 | 9 |
TCGT | TRUE | 1 | 9 | 9 | 12 |
TGGA | TRUE | 1 | 4 | 14 | 8 |
TGGG | TRUE | 1 | 4 | 5 | 8 |
AGGG | TRUE | NA | 5 | 4 | 5 |
CCGT | TRUE | NA | 5 | 5 | 14 |
GGTG | TRUE | NA | 5 | 7 | 10 |
GTGC | TRUE | NA | 5 | 3 | 6 |
AGCG | TRUE | NA | 4 | 6 | 7 |
CCGA | TRUE | NA | 4 | 4 | 9 |
CCTG | TRUE | NA | 4 | 6 | 13 |
CTGG | TRUE | NA | 4 | 7 | 17 |
GTCG | TRUE | NA | 4 | 6 | 12 |
GTGG | TRUE | NA | 4 | 3 | 10 |
CCCA | TRUE | NA | 3 | 2 | 9 |
CCGG | TRUE | NA | 3 | 2 | 9 |
CGAG | TRUE | NA | 3 | 3 | 11 |
CGCG | TRUE | NA | 3 | 2 | 8 |
CGTC | TRUE | NA | 3 | 7 | 8 |
CGTG | TRUE | NA | 3 | 4 | 3 |
GAGG | TRUE | NA | 3 | 5 | 10 |
GCAG | TRUE | NA | 3 | 3 | 9 |
GCTG | TRUE | NA | 3 | 6 | 13 |
GGGA | TRUE | NA | 3 | 5 | 11 |
GGTC | TRUE | NA | 3 | 7 | 10 |
TCCC | TRUE | NA | 3 | 9 | 14 |
TGGC | TRUE | NA | 3 | 4 | 9 |
CAGG | TRUE | NA | 2 | 2 | 8 |
CGGG | TRUE | NA | 2 | 3 | 15 |
GGCT | TRUE | NA | 2 | 6 | 13 |
TGCC | TRUE | NA | 2 | 8 | 6 |
CCCG | TRUE | NA | 1 | 2 | 9 |
CCGC | TRUE | NA | 1 | 8 | 10 |
CGGC | TRUE | NA | 1 | 8 | 7 |
GCCC | TRUE | NA | 1 | 5 | 10 |
GCCG | TRUE | NA | 1 | 6 | 7 |
GCGG | TRUE | NA | 1 | 1 | 7 |
GGCG | TRUE | NA | 1 | 5 | 8 |
GGGG | TRUE | NA | 1 | 7 | 14 |
CGCA | TRUE | NA | NA | 12 | 10 |
ACCC | TRUE | NA | NA | 6 | 13 |
GCGC | TRUE | NA | NA | 2 | 9 |
It may be helpful to visualize the k-mer count distributions to get a better feel for the similarities and differences between the sequences.
Bar plots of count distributions for each experiment
#Function:
#create bar plots with bar_plot()
bar_plot <- function(tbl) {
tbl %>%
ggplot(aes(kmer_count, kmer, fill = kmer_count)) +
geom_col() +
scale_fill_viridis_c() +
theme(legend.position = 'none') +
theme(axis.title.y = element_blank(),
axis.text.y = element_blank(),
axis.ticks.y = element_blank()) +
labs(x = "K-mer counts")
}
p1 <- fasta_df$sequence[1] %>%
kmer_len() %>%
bar_plot() +
labs(title = 'Exp1 kmer counts')
p2 <- fasta_df$sequence[2] %>%
kmer_len() %>%
bar_plot() +
labs(title = 'Exp2 kmer counts')
p3 <- fasta_df$sequence[3] %>%
kmer_len() %>%
bar_plot() +
labs(title = 'Exp3 kmer counts')
p4 <- fasta_df$sequence[4] %>%
kmer_len() %>%
bar_plot() +
labs(title = 'Exp4 kmer counts')
(p1 + p2) / (p3 + p4)
Notably, the count distributions are progressively less varied.
Summary table
The max count
and sd count
variation are worth exploring further.
#create a summary table with the median, min and max
sequences %>%
group_by(experiment) %>%
drop_na() %>%
summarize(`median count` = median(kmer_count),
`mean count` = round(mean(kmer_count)),
`sd count` = round(sd(kmer_count)),
`max count` = max(kmer_count)) %>%
gt() %>%
tab_header(title = md("**K-mer counts summary table**"),) %>%
tab_options(
heading.background.color = "#EFFBFC",
table.width = "75%",
column_labels.background.color = "black",
table.font.color = "black") %>%
tab_style(style = list(cell_fill(color = "Grey")),
locations = cells_body())
K-mer counts summary table | ||||
---|---|---|---|---|
experiment | median count | mean count | sd count | max count |
Exp1_counts | 5 | 11 | 15 | 77 |
Exp2_counts | 7 | 10 | 9 | 48 |
Exp3_counts | 9 | 10 | 5 | 28 |
Exp4_counts | 10 | 10 | 3 | 17 |
Density plots
We can get a better feeling for the count distributions with density plots.
sequences %>%
group_by(experiment) %>%
ggplot(aes(kmer_count, fill = experiment)) +
geom_density() +
facet_wrap(~experiment) +
scale_fill_viridis_d() +
theme(legend.position = 'none') +
labs(title = "Density distributions of k-mers (4-mers) by count",
x = "Kmer count",
y = "Density")
Binning the kmer counts for a more general pattern
In the next two plots, the k-mer counts are grouped into 8 categories (10-80 k-mer counts by 10 and an other category). For all experiments, most of the 256 possible k-mers appear less than 10 times. So as not to dwarf the k-mer counts that appear more than ten times, the ‘10 and under’ category is shown separately.
sequences %>%
mutate(kmer_count = as.numeric(kmer_count),
counts_bins40_200 = case_when(
kmer_count <= 10 ~ as.character('10 and under'),
between(kmer_count, 11, 20) ~ as.character('11-20'),
between(kmer_count, 21, 30) ~ as.character('21-30'),
between(kmer_count, 31, 40) ~ as.character('31-40'),
between(kmer_count, 51, 60) ~ as.character('41-50'),
between(kmer_count, 61, 70) ~ as.character('41-50'),
kmer_count >= 71 ~ as.character('71 and greater'),
TRUE ~ 'other'
)) %>%
filter(counts_bins40_200 == "10 and under", counts_bins40_200 != '11-20') %>%
group_by(counts_bins40_200) %>%
ggplot(aes(counts_bins40_200, fill = experiment)) +
stat_count() +
scale_fill_viridis_d() +
labs(title = "Count of binned k-mers",
subtitle = "The distribution of k-mer counts between 0-10",
x = "K-mer count",
y = "Density")
sequences %>%
mutate(kmer_count = as.numeric(kmer_count),
counts_bins40_200 = case_when(
kmer_count <= 20 ~ as.character('10 and under'),
between(kmer_count, 11, 20) ~ as.character('11-20'),
between(kmer_count, 21, 30) ~ as.character('21-30'),
between(kmer_count, 31, 40) ~ as.character('31-40'),
between(kmer_count, 51, 60) ~ as.character('41-50'),
between(kmer_count, 61, 70) ~ as.character('41-50'),
kmer_count >= 71 ~ as.character('71 and greater'),
TRUE ~ 'other'
)) %>%
filter(counts_bins40_200 != "10 and under", counts_bins40_200 != 'other') %>%
group_by(counts_bins40_200) %>%
ggplot(aes(counts_bins40_200, fill = experiment)) +
stat_count() +
scale_fill_viridis_d() +
labs(title = "Count of binned k-mers",
subtitle = "The distribution of kmer counts between 10-80",
x = "K-mer count",
y = "Density")
We see that experiment 2 dominates k-mer counts in the 30-40 range and experiment 1 dominates in the greater than 40 range.
Instructions: k-mer counting script for the command line
To help you check for imbalanced k-mer distributions in future experiments, I designed a k-mer counting analysis script that works from the command line. The k-mer counter let’s you input a FASTA file and k-mer length, and returns a tab separated file of k-mer counts ordered by frequency. The program also returns messages about the analysis including:
- Input sequence length
- K-mer length
- Which nonstandard nucleotides the program can identify
- Whether the k-mer length is acceptable for the sequence range
- The top 10 k-mers by count
- Whether the given sequence contains standard or nonstandard nucleotides. The nonstandard nucleotide warning can be tested with the following file: takehome/challenge1/nonstandard_nucs.fasta
To use the k-mer counting tool:
1. Download the directory I sent you and open it in the command line
2. Change the permissions for kmer_counter_tool.R script to make it executable on your machine (chmod +x kmer_counter_tool.R)
3. Then run the following incantation in your command line (with custom input and output file names):
Rscript kmer_counter_tool.R ‘input_file’ kmer-length –output_file ‘output_file’
e.g.,
4. See the image below for the expected output in the command line (Note: I am working on a mac).
When running the script, a new output file (tab separated format) with k-mers ordered by frequency is generated.